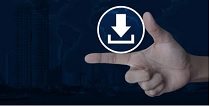
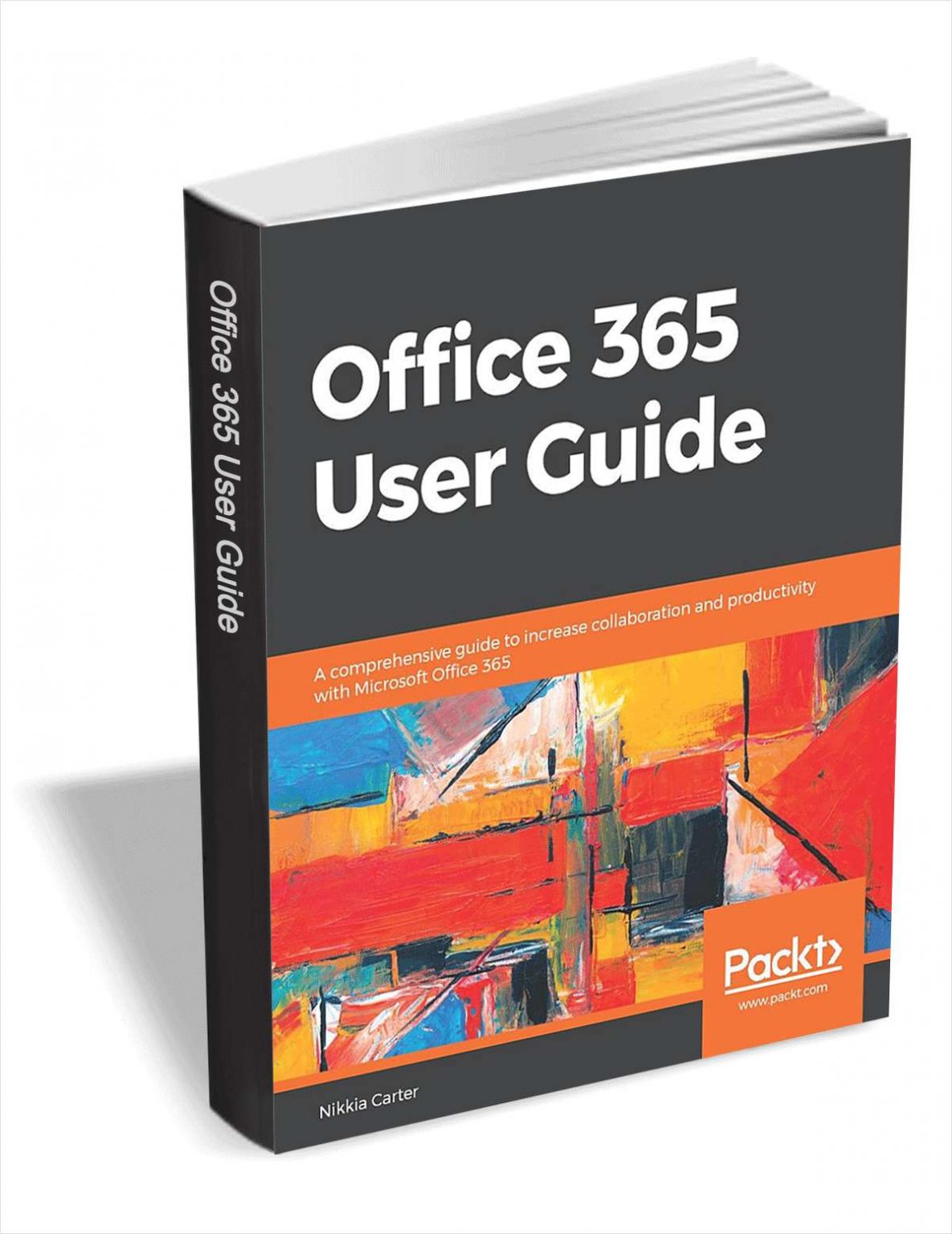
- #SYNCOVERY USER MANUAL SERIAL#
- #SYNCOVERY USER MANUAL MANUAL#
- #SYNCOVERY USER MANUAL FULL#
- #SYNCOVERY USER MANUAL SOFTWARE#
Probability Map Viewer provides an interactive interface through which the image can be explored using Google Maps-like controls, such as panning.
#SYNCOVERY USER MANUAL FULL#
Once complete, the full image is displayed seamlessly in the Probability Map Viewer web application via an embedded Jetty web server that is run from the local machine ( Supplementary Figs S2 and S3). Probability Map Viewer automatically monitors the data server’s collection path, and, with the help of IMOD command line tools ( Kremer et al., 1996), converts each full SBEM raster into a set of square tiles in the PNG image format. The Probability Map Viewer instance is initialized from the command line of a local Linux machine. The most recently synced data are stored in the SSD cache of the data server to ensure rapid accession.Ģ.2 Visualization and NRT probability map generation Münster, Germany), which runs at the lowest priority on the acquisition computer and checks for new image files in a collection-specific path every five seconds.
#SYNCOVERY USER MANUAL SOFTWARE#
The transfer of newly collected images to the data server is facilitated by the Syncovery file synchronization software (Super Flexible Software Ltd. Both the acquisition computer and data server use RAID 5 storage with an SSD cache for increased performance and redundancy. To prevent disruption of a live imaging run, newly collected data are rapidly transferred from the acquisition computer to a Windows 7 data server via a 10G Ethernet connection ( Supplementary Fig. A data acquisition computer running Windows 7 and the DigitalMicrograph (DM) software (Gatan, Inc.) controls the acquisition and stores all output images in the DM (.dm4) format. Our SBEM imaging system consists of a Gatan 3View (Gatan, Inc.) incorporated into a Zeiss Merlin scanning electron microscope (Zeiss). Probability Map Viewer allows users to select one or more VCs, apply a subset of them to collected data, and assess their performances on the fly, long before the acquisition is complete. To address these limitations, we developed Probability Map Viewer, a web-based visualization and processing tool that computes and displays automated VC probability map results in near real-time (NRT) during active SBEM collections. Furthermore, under the current paradigm, segmentation decisions are typically made only after the full dataset has been acquired, resulting in a significant delay between data collection and the quantitative analysis of biological data.
#SYNCOVERY USER MANUAL MANUAL#
Though the time required to generate such training sets pales in comparison to that of manually segmenting the entire volume, it is not insignificant some use cases require days of manual effort to produce training sets without guarantee that the resultant VCs will prove viable.

Supervised algorithms, which train voxel classifiers (VCs) by learning features based on a supplied set of training data, have proven optimal for tackling the challenges posed by densely stained EM images ( Liu et al., 2014). To address this bottleneck, a number of approaches for automated segmentation have been developed ( Peddie and Collinson, 2014). Since the man-hour cost of manual segmentation often scales in a linear manner with dataset size, it does not represent a sustainable solution for annotating the teravoxels of image data now being produced daily by automated 3D EM platforms. Such analyses, however, are critically dependent upon the accurate delineation of structures of interest via robust segmentation routines.
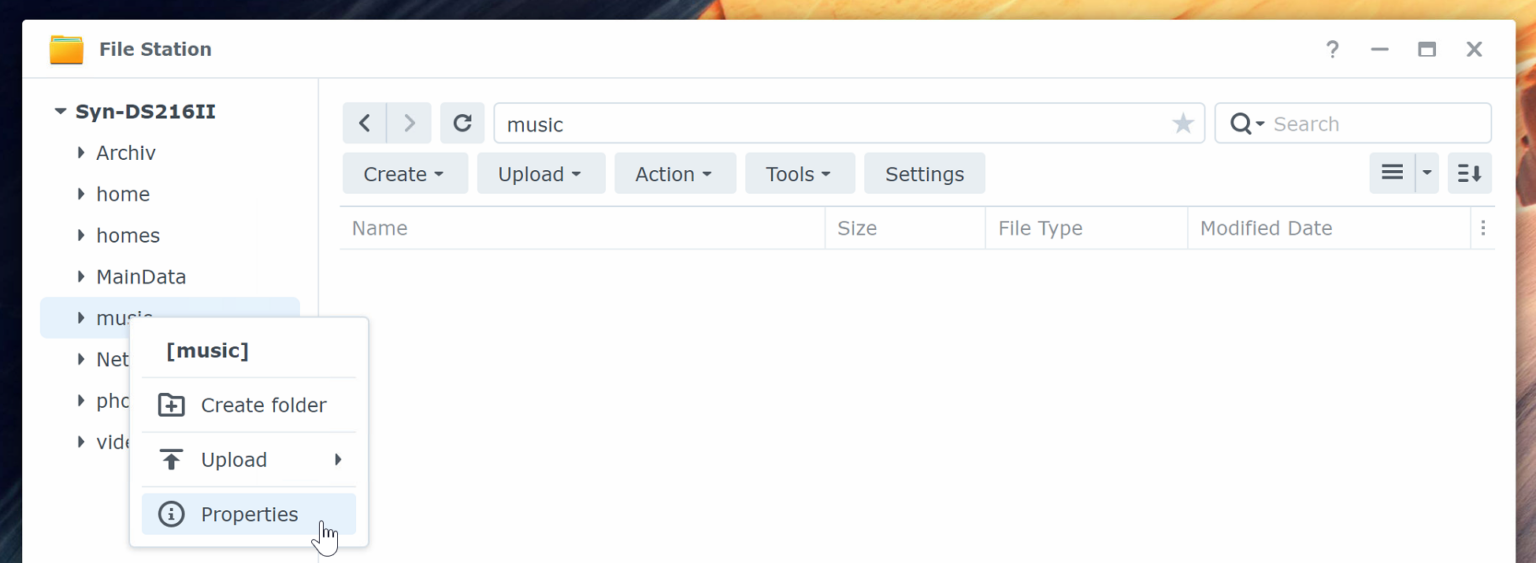
In the neurosciences, such data are being leveraged to map neuronal wiring diagrams ( Kasthuri et al., 2015) and quantify the morphology, distribution and state of organelles ( Perez et al., 2014).
#SYNCOVERY USER MANUAL SERIAL#
Automated 3D electron microscopy (EM) platforms, such as serial block-face scanning EM (SBEM) ( Denk and Horstmann, 2004), are capable of producing expansive volumes of data at resolutions sufficient for visualization of organelles and certain macromolecular complexes.
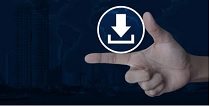